Chapter 5 Resampling Methods
Learning objectives:
Big picture - to learn about 2 resampling methods: cross-validation and the bootstrap
- Both refit a model to samples formed from the training set to obtain additional information about the fitted model: e.g. they provide estimates of test-set prediction error and the standard deviation and bias of parameter estimates.
Recall that the training error rate is often quite different from the test error rate and can dramatically underestimate it
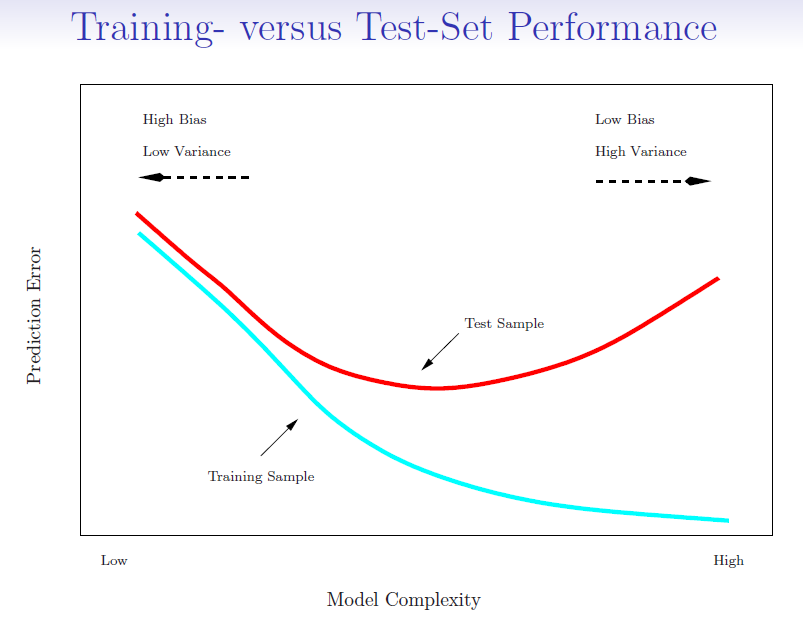
Figure 5.1: Training- versus Test-Set Performance. Source: https://hastie.su.domains/ISLR2/Slides/Ch5_Resampling_Methods.pdf
Best solution is to have a large test set (which is not often available)
Some methods (AIC, BIC) make a mathematical adjustment to the training error rate in order to estimate the test error rate.
Here we consider methods that estimate the test error by holding out a subset of the training observations from the fitting process. Then applying the statistical learning method to those held out observations
We will:
- Use a validation set to estimate the test error of a predictive model.
- Use leave-one-out cross-validation to estimate the test error of a predictive model.
- Use K-fold cross-validation to estimate the test error of a predictive model.
- Use the bootstrap to obtain standard errors of an estimate.
- Describe the advantages and disadvantages of the various methods for estimating model test error.